From Data Lakes to AI: An Interview with Invert’s Head of AI
.png)
Suhas Guruprasad, the Head of AI at Invert, dives into the evolution of artificial intelligence, distinguishing between the actual capabilities of AI and the often exaggerated hype surrounding it. He also explores the potential implications and transformative effects of AI on the bioprocessing industry.
You were the Head of Engineering at Zalando, tell me about your role there. What is one thing you built that you’re proud of?
Suhas: I joined as a Senior Engineer in 2017 and was later promoted to management roles. Initially, my focus was on Data Lake and Big Data technology. I was part of the team that wrote Data Lake v1 at Zalando. It had the capability to process, transform and ingest Terabytes of data in real time!
I was always both a mathematician and a computer scientist at heart, so I have had an attraction to AI since as long as I know. In school, I’d build several toy apps that tried to mimic intelligence, but failed each time of course. Now I realize how far we’ve come in terms of AI as a society. Primarily due to the exponential reduction in data storage costs and exponential increase in computing power.
At Zalando, I built the team that created one of the first ML platforms in Europe. We had a working end-to-end ML platform all the way back in 2018… that feels so far away now. When I left in late 2022, there were hundreds of ML models trained and deployed every single day on the platform, safely and securely. We reduced the lead time to shipping AI products by months, or in some cases years. The lever that it created was massive.
What made you decide to join Invert?
Suhas: When I joined Invert, I’d been working in e-commerce for over 7 years and, by then, running a large organization. I definitely wanted to try something radically new. I guess I was looking for a combination of building something with my own hands and running a team.
I was talking to several founders, mostly early stage start-ups, both in Fintech and Biotech. I’m actually quite passionate about Fintech, so I was very sure I’ll end up in one. But luck has it otherwise, and how lucky I’ve been.
In my first conversations with both Martin and Holger, I remember coming out of it being absolutely impressed with them. I sensed how smart and passionate they were, and thought that I’d definitely want to be around them.
Ultimately, I’m an accelerationist at heart. Biology and its applications are so tangible and magical at the same time. Being able to deploy technology to accelerate the development of life changing products such as therapeutics, food, and materials is something that I’m so lucky to be able to do.
Has your lack of a biology background been a challenge at all, or is it also a strength?
Suhas: It definitely can be a challenge. I certainly believe domain knowledge is essential to building great products. So right now I’m on a mission to study as much biology as possible in as little time as possible.
Having a vision is only possible when you really know your field inside and out. You know it so well that you’re able to see the future. Although I’m not yet an expert in bioprocessing, Invert is filled with people who are. So we’re really able to lean on each other to develop solutions that address the evolving needs of bioprocess scientists and engineers.
Also, to my delight, a lot of the literature coming out of academia is closer to computer science than I expected. I read a lot of these papers, and they’re all talking about things like PCA, Neural Nets, Hybrid models, data quality and cleanliness, Bayesian thinking and all those usual topics that one would find in a good computer science college course.
The additional advantage I believe I have is close to 15 years of shipping software products. So, I come with an absolute focus to ship software for the biotech industry.
AI can be a bit ambiguous – what excites you about that tech? How has it been over-hyped?
Suhas: Most of the AI conversations today are around LLM’s or Large Language Models.
If you look a bit at AI history since the 1950s, we have experienced high and low seasons – we call them summers and winters. The wikipedia article on AI winter is actually great and I recommend reading it. We’re typically in 10-year hype cycles with AI and currently, we’re in the hottest of summers. Since the 2010’s, a series of neural network architectures have taken us by storm and perform quite well on various tasks.
The latest is something called a transformer network, which improves on recurrent networks (RNNs) and long short-term memory networks (LSTMs). Google Translate, for instance, switched to LSTMs back in 2016 and the team had achieved the same performance in 9 months that the previous team had taken 10 years to do.
So essentially a bunch of people from Google figured out a magic trick in 2017. They called this “attention”, like in the same context as, “hey, pay attention”. Forbes actually has a good article on this for the casual reader called, “Transformers revolutionized AI. What will replace them?”. What we see today, for example with ChatGPT, is stemming from this little magic trick.
If you’d asked me 2 years ago what AI is, I would’ve told you that AI is just a marketing term. You know, it might actually be different today.
It’s all so dramatic in AI right now. If you’re following the conversation these days, there are two camps emerging: the accelerationists, led by Marc Andreessen, Yann LeCun, and Andrew Ng; and the doomsayers, including Elon Musk, Eliezer Yudkowsky, and AI pioneer Geoff Hinton who is often called the Godfather of AI. Imagine, the Godfather of AI calling for a stop on AI research.
The accelerationists say we should keep going, and do even more with AI. While the doomsayers are calling a stop to all AI development. Although, recently we have seen a complete reversal from Elon Musk with the release of Grok, a competitor to ChatGPT.
Some of the hype is well deserved — we have for the very first time in history passed many Turing test equivalents. GPT-4 has passed several human-level exams, like the Bar exam with 298/400, LSAT with 163, SAT with 700/800, GRE with 163/170. These are all impressive numbers.
So in that sense, everything about AI is exciting right now. It’s a melting pot of political, economical, technological and anthropological ideas, all happening in real time, at warp speed.
What are some of the ways you see AI/ML playing a role in biotechnology R&D and MFG in the next 5 years?
Suhas: I have to obviously start by mentioning Copilot, which we just shipped to all of our customers. With Copilot, people are able to interact with bioprocess data in natural language, just like they would with ChatGPT. One of our internal conversations was to see how easy it would be for scientists to just think of a question, ask that out loud to Invert, and Copilot creates a set of analyses using large amounts of contextualized bioprocess data. No coding involved. It’s a definite UI/UX breakthrough for data exploration, and very relevant for speeding up experiments as well as removing the barrier between users and their data.
The more exciting thing that comes to mind is the proliferation of models like AlphaFold and AlphaMissense. So I’m following the work of Isomorphic Labs religiously. They’re that DeepMind spin off company which now focuses only on Biology problems, with a special focus on AI for drug discovery.
If you step back and look at information manipulation as the primary lens, biology is probably running a couple decades behind. We were manipulating bits with vacuum tubes as early as the 1900’s, though semiconductors came much later. In contrast, things like recombinant DNA came out in the late 1970’s. So one can look at the arc of computer science to look for clues on what’s possible with manipulating information once the power to manipulate is there.
The real challenge though, and I can’t stress this enough, would be to productize and ship AI so that it’s applied to the right problem at the right time. Ideas in AI/ML have existed for a while, but productizing it, and making it available on top of any data size, and at any scale, will be a key differentiator. Those who are able to do this will pull ahead, and those who don’t will fall back.
What are some of the challenges of applying AI/ML to bioprocess data?
Suhas: The first thing that comes to my mind is data quality. I often see poorly organized lab sheets where data is spread across different sheets, workbooks, you name it. Time measurements are not synchronized. Missing data. Unstructured data. All sorts of issues with data quality. At Invert, we actually call this the 6s problem. But to be fair, data quality is an issue in every industry vertical — anywhere there are humans!
It’s important to note that all of modern deep learning is possible due to massive amounts of labeled data. A model is only as good as the quality of data that you have, “garbage in, garbage out” as the saying goes.
One of the things that we’re doing at Invert is figuring out how much we can remove that human-in-the-loop. It’s funny that I think about this. In Reinforcement learning, human-in-the-loop is so useful as a rewarding mechanism for AI, but for structured data curation, it’s best humans are not there at all. So we’re writing these agents that directly read measurements from bioreactors. No lab sheets!
We’ve come a long way on this by already supporting some of the major reactors in the market like Sartorius, Eppendorf, Solaris, and many others. I think that’s really exciting to me – by having a real-time stream of high-quality bioprocess data directly from the reactors, across various scales, imagine the possibilities. Things like automatic anomaly detection with recommended interventions, mid-run performance predictions, and even intelligent process control and scale-up.
The other macro challenge I see is in what I call world modeling. For instance, in e-commerce when you’re predicting sales, or in stock markets when you’re predicting stock price, you consider some world model. A model with world events, news, weather etc. That is always a challenge. You never really know what’s really going to happen in the future.
Just like world modeling at the macro-level, there are a lot of similarities when modeling at cellular level within a bioreactor environment. I see some advances with metabolic modeling, for instance the CHO Simulator post recently published by Asimov. That really is quite exciting.
What are you most looking forward to building at Invert or is there a particular project you’re excited about?
Suhas: The obvious cop out answer is everything! I really feel excited about the momentum that we have. We’re also not doing any tricks. We’re not an “Uber-for-X”. We’re a bunch of smart people building software for biomanufacturing. This, as a premise to me, when put in context on how this industry vertical is set to grow, is kind of mouth watering.
If you want me to pick something in particular, then I’d say I’m really excited about building all kinds of process intelligence into our offering. Think about it. We automatically get data in. We visualize it beautifully. Now, we also provide intelligence and guidance on the processes. That’s a whole productivity package. The perfect biomanufacturing software platform.
It also completes my love story with productivity tooling and reducing lead time. I want biologists to ship their product much faster than the status quo today. It’s the accelerationist mindset.
What advice would you give to biotech companies who are interested in using ML/AI/Data Science to further accelerate their development?
Suhas: Talk to Alex about Invert!
Right, so one of the biggest mentoring challenges that I faced at Zalando was helping team leads make the right tooling decision and picking the right tech stack for their product. There was always a build vs buy battle — should I build something myself or buy something someone has already made?
It’s actually coming down to a bunch of variables — skills and talent pool, dollar amount left in the bank, product maturity, senior management vision and so on. I also really like the concept of the Idea Maze, by Marc Andreessen here. If you’re a leader, you should really know what decision to take next, because you’ve kind of exhausted all other possible ideas in the idea maze. So in that context, I think this is leadership advice. Good leadership is half the job.
No matter whether or not you plan on investing in AI either internally or externally, make sure you are always maintaining clean and contextualized (labeled) data. It will help your efforts today, and also provide you with that foundation in the future if and when you decide to deploy AI.
All else aside, if there’s one key takeaway advice I have to give, it would be this — AI is here, and it’s real. The world’s smartest and most talented people are jumping on the AI wagon and trying to find every nail to hit with this Thor’s hammer.
Before you know it, someone might disrupt you. Therefore, approach AI with a heightened sense of urgency. That would be my advice.
.png)
How Invert uses meta-learning to leverage old bioprocess data
With meta-learning techniques, Invert’s ML team was able to reduce new data needs by as much as 90% by leveraging old data from different, but loosely related, bioprocess programs.
Read More ↗.png)
Pioneers in Bioprocessing: Q&A with Biopharma Executive, Steven Lang, Ph.D.
Biotech and synthetic biology companies have much to do. Handling everything in-house—from strain design to process development—can stretch funding and resources thin.
Read More ↗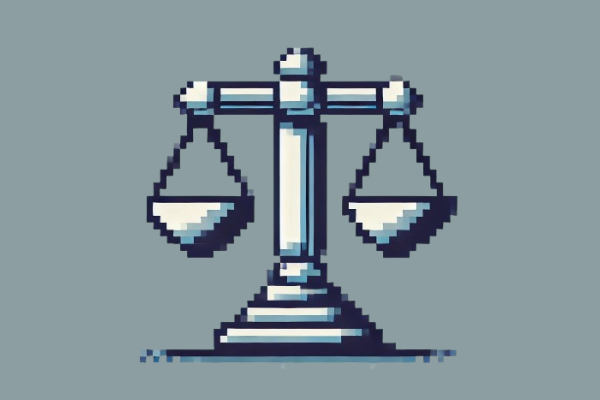
A Guide to Build vs Buy: Biotech Software Edition
For biotech and synthetic biology companies, there’s always A LOT of work to do. As these companies quickly learn, trying to handle everything in-house–from strain design and high-throughput screening to process development and beyond–can spread funding and resources thinly.
Read More ↗