How Invert uses meta-learning to leverage old bioprocess data
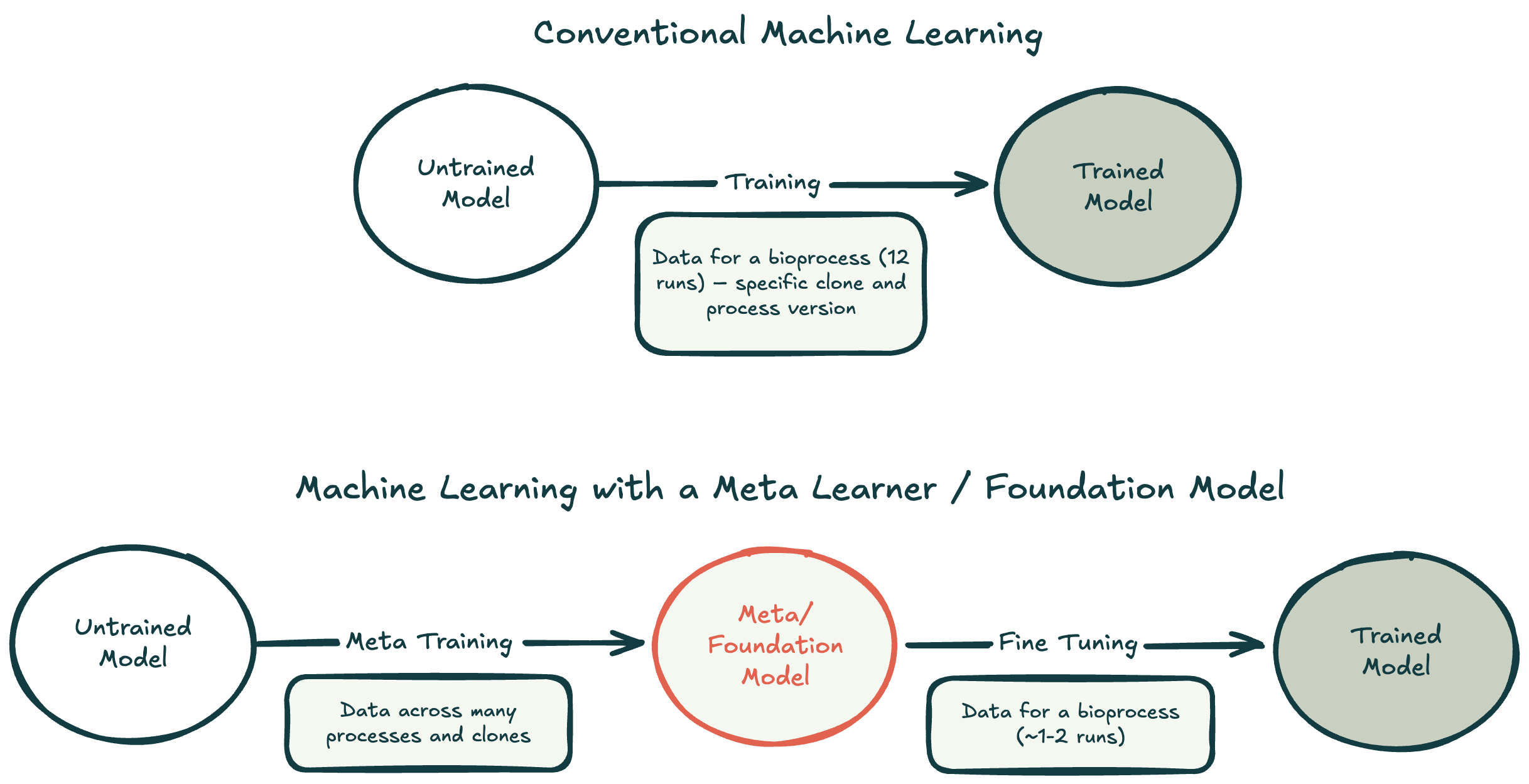
Imagine launching a new drug program and already having 90% of your process development figured out. With meta learning, this is a real possibility – and this is a major focus for us at Invert.
Our initial results in this product area are very promising. They suggest that for new projects, biomanufacturers can leverage older data (from different, but loosely related projects) to cut the need for new data by as much as 90%.
The high cost of generating new bioprocess data
In biomanufacturing, generating new data is very expensive – so we are very excited about this early breakthrough.
Relatively small batches (1-20L) can cost on the order of $10,000 to $100,000 per batch, depending on complexity. Pilot-scale batches (50-500L) can reach $50,000 to $500,000 per batch, and in some cases, even higher.
In addition to these hard costs, the value of speed (or, framed in the negative, opportunity cost of moving slowly) is immense. Biomanufactured products require a great deal of specialized labor, and involve opportunity costs associated with the use of expensive facilities and time-to-market.
An example: leveraging data from previous drug programs
Consider a major pharmaceutical company that specializes in monoclonal antibody-based therapies. They may have dozens of completed drug programs with valuable data stored in various filesystems and databases.
The cost of generating data for a single program is typically in the range of $50-200M in hard costs – so for a dozen such programs, the total cost including variable costs, capital costs, and labor is staggeringly high.
When this pharmaceutical company develops a new drug program, process development scientists lean on intuitions (developed from previous work) about how best to design future experiments. But old data is rarely used to inform next experiments, especially with machine learning.
Our early product work at Invert suggests that this old data – combined meta-learning techniques and hybrid models – can reduce the new data needs in new drug programs by as much as 90%. This translates to savings of tens of millions of dollars in hard costs, and dramatically faster time-to-market.
Our early work in meta-learning at Invert
We work with a range of customers at Invert, including Contract Development and Manufacturing Organizations (CDMOs) who service the bioprocessing needs of many clients. Often these CDMOs will specialize in a host organism or in a product area. Invert caters to CDMOs helping them manage their bioprocess data across all their different projects and clients.
For predicting bioprocesses, we found that hybrid models work exceptionally well. They are founded upon some physical model, for example, ordinary differential equations that describe the dynamics of cell growth or product formation. The physical model is wedded to a black box approach such as a neural network, which provides adaptability to a variety of input data. Hybrid models are also amenable to meta-learning, where we first train a meta model that can be adapted for different projects.
.png)
So far we’ve seen that with meta-learning approaches on a single CDMO customer, we can reduce the number of data needed by as much as 90% while maintaining 90% of performance as they embark on new projects.
Our clients are using models to design experiments and to help accelerate development on new projects. In the long run, we want to use the models to help with process monitoring and eventually self-driving their process to maximum performance.
Why aren’t more biomanufacturers leveraging old data?
Isn’t it obvious to leverage previous data to guide new experiments in a more explicit, ML-oriented way? It is easier said than done, and there are a few reasons for the current state of affairs:
- Meta-learning is advancing rapidly, with major breakthroughs in recent years. Industry adoption is still a fairly recent phenomenon.
- Data is inaccessible. For this data to be used in AI/ML applications, machine learning scientists must be able to access it. Today, this data is typically distributed across various file systems, databases, and hardware.
- Data is not standardized. As the saying goes, when it comes to AI/ML, it’s garbage in, garbage out. It’s common for this data to be in a bad state, with inconsistent labels, poor context, outliers, errors, and other issues.
As an aside: we operate with an unfair advantage at Invert (and so do our clients), because our product automatically cleans, standardizes, and centralizes bioprocess data. As such, our internal AI/ML team has the benefit of working with clean data that is ready for modeling and meta-approaches.
We expect that pharmaceutical companies and synthetic biology companies will prioritize data preparation and accessibility in the coming years, either using products like Invert or through internal initiatives.
With AI-ready data in hand, we expect more biomanufacturing companies to unlock the value of their historical data in the future and to develop bioprocesses faster and cheaper.
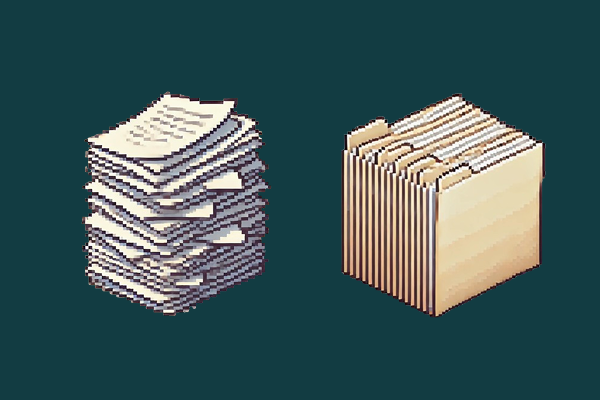
Why ELNs and LIMS are not enough for PD teams
One of the questions we’re asked frequently is whether Invert is an ELN or LIMS—our answer is that Invert captures both systems’ strengths and fixes their weaknesses. We’ll discuss the trade-offs and shortcomings of ELN and LIMS when it comes to bioprocess data to explain how.
Read More ↗.png)
Pioneers in Bioprocessing: Q&A with Biopharma Executive, Steven Lang, Ph.D.
Biotech and synthetic biology companies have much to do. Handling everything in-house—from strain design to process development—can stretch funding and resources thin.
Read More ↗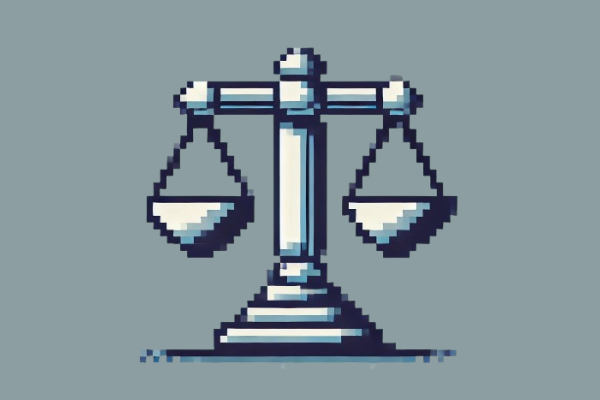
A Guide to Build vs Buy: Biotech Software Edition
For biotech and synthetic biology companies, there’s always A LOT of work to do. As these companies quickly learn, trying to handle everything in-house–from strain design and high-throughput screening to process development and beyond–can spread funding and resources thinly.
Read More ↗